Algorithms increase the efficiency for researchers working on battery aging estimations. “Unlike previous studies that required collecting, storing, transmitting, and processing a large dataset, the team only needed a small dataset that could be easily and directly stored in electric vehicles”, says Assoc. Prof. Changfu Zou, at Chalmers.
In collaboration with CEVT AB, the Chalmers team, involving Assoc. Prof. Changfu Zou, Lic. Yizhou Zhang (Industrial PhD student), and Prof. Torsten Wik, has developed diagnostic and prognostic algorithms for battery aging that can accurately estimate battery state of health and predict the future aging trajectory and remaining useful life.
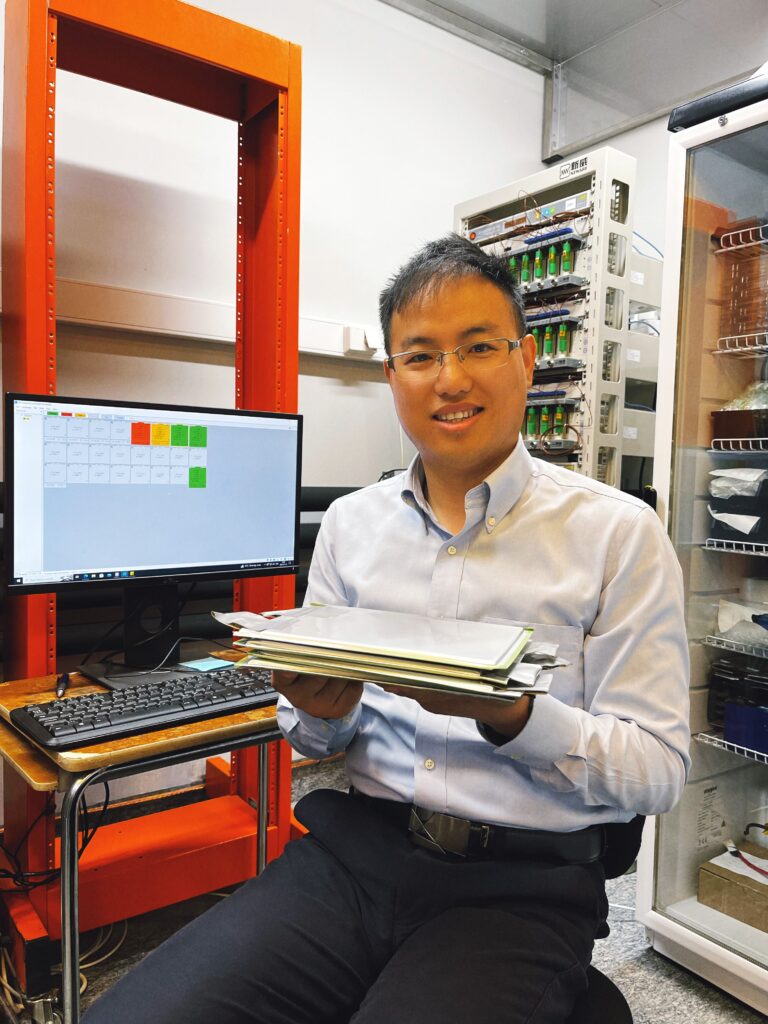
“What sets this research apart is its applicability, accuracy, and practicability. Unlike previous studies that required collecting, storing, transmitting, and processing a large dataset, the team only needed a small dataset that could be easily and directly stored in electric vehicles”, says Assoc. Prof. Changfu Zou.
The algorithms also work accurately and efficiently under arbitrary real-world usage, making them applicable to a wider range of situations. Additionally, the algorithms learned from an electric vehicle fleet can be applied to new vehicles through a combination of offline and online learning, and the data can be in any format of time series and histograms. The team validated their algorithms using data from over 7000 real-world electric vehicles, proving their effectiveness.
This research was funded by the Swedish Energy Agency under the FFI Program and is part of the “Data driven battery aging prediction” project. We are excited to see how this research can help improve the longevity of batteries in electric vehicles, ease their maintenance, and increase the second-life value for a circular economy. We look forward to seeing more developments in this field.
If you are interested in learning more, we encourage you to check out the team’s recent publications listed below:
[1] Zhang, Y., Wik, T., Bergström, J., Pecht, M., & Zou, C. (2022). A machine learning-based framework for online prediction of battery ageing trajectory and lifetime using histogram data. Journal of Power Sources, 526, 231110.
[2] Zhang, Y., Wik, T., Bergström, J., & Zou, C. (2023). State of health estimation for lithium-ion batteries under arbitrary usage using data-driven multi-model fusion. IEEE Transactions on Transportation Electrification.
[3] Zhang, Y., Wik, T., Huang, Y., Bergström, J., & Zou, C. (2023). Early prediction of battery life by learning from both time-series and histogram data. IFAC World Congress, Yokohama, Japan.