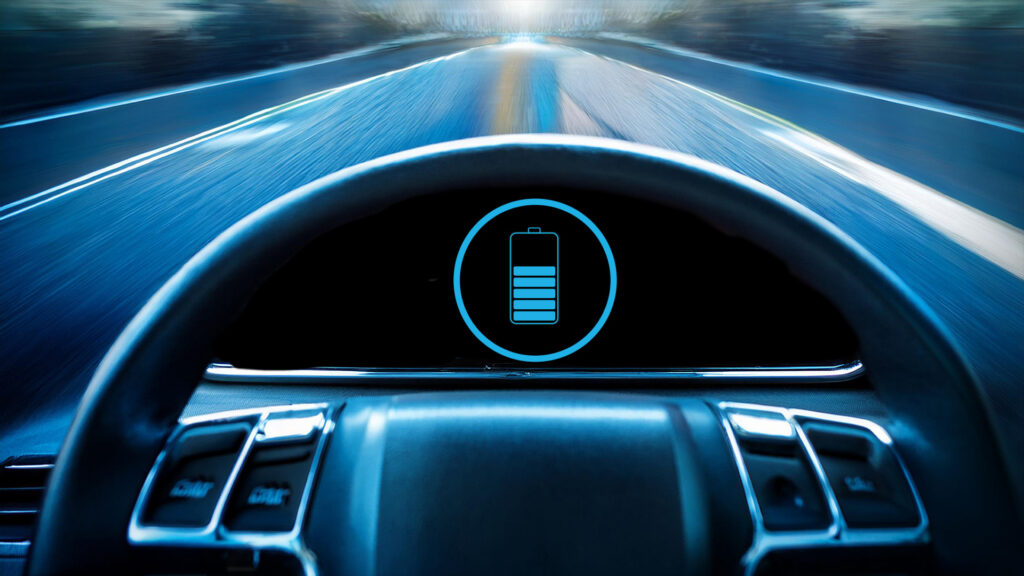
The acceptance of electrical vehicles is dependent on long travels being time competitive and predictable. Range anxiety and fear of queuing at charging stations are serious concerns for switching to electrical vehicles. “Charge” is a project developing a novel intelligent trip planning system that can save energy, reduce total trip time and remove unpredictable factors that can cause unease for drivers and passengers.
Current online trip planners provide rather simple information like locations of charging stations and estimated charging time. These planners presume that fast charging is available immediately and that the vehicle battery is at optimal temperature range, which often is not the case. By incorporating historical data, intelligent control strategies, predictive information from vehicle to vehicle and vehicle to infrastructure communication, it’s possible to create a more intelligent trip planner.
Novel overall approach
E-mobility trip optimization is mainly related to four sub-areas. The onboard energy management system, the thermal management system, the eco-driving system, and coordination of electricity charging. The “Charge” project does not propose new structural solutions in each individual sub-area, instead it takes a novel holistic approach.
“This holistic approach is rather new and is anticipated by the industry. Trip planners today are very basic and can be improved a lot by developing stochastic models from historical data. Such models can then be used to make predictions about chargers and power availability at the stations. If we also combine the stochastic models with real-time communication between vehicles and charging stations, the predictions can become even more accurate,” says Lorenzo Montalto, PhD student at Chalmers University of Technology.
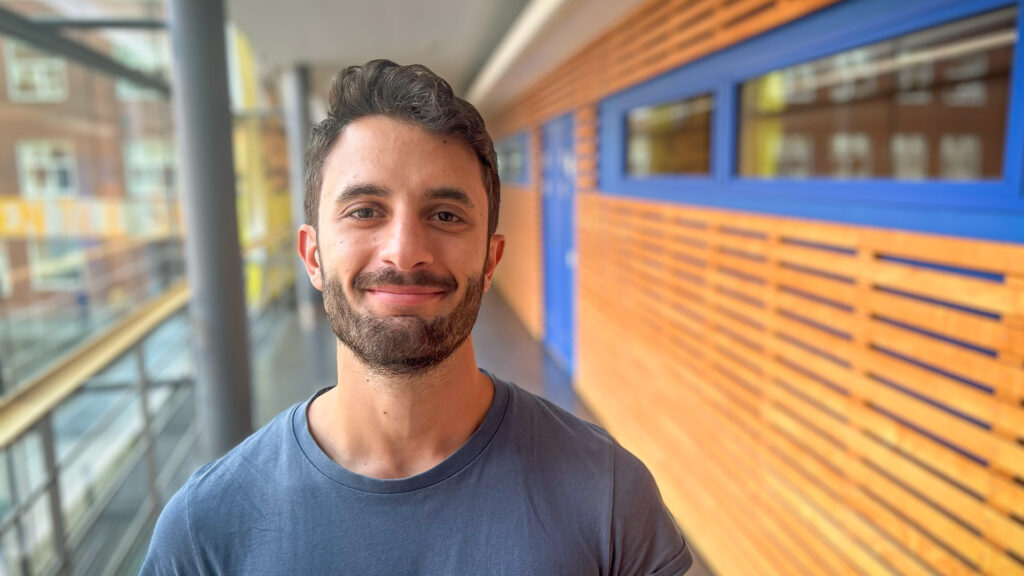
Simulated driving scenarios using real-car navigation system
Augmented reality experiments will be conducted using the real-car navigation system with a simulated scenario for a fleet of ten vehicles driving from Gothenburg to Uppsala. The project will combine real-time eco-driving, energy and thermal management from the vehicle perspective, and charging from both the vehicle and infrastructure perspective.
Stochastic models will be developed on anticipated distribution of charging cost, power availability, waiting time and overstay cost at charging facilities. Open-source tools will be used as a proof of concept for the initial development and for most of the published results. The final algorithms that are meant to run onboard the vehicles will be custom developed and will likely not be open source.
91 percent reduction in computational execution time
“From a technical point of view, I think it’s possible to see these intelligent planners implemented in just a few years from now. Lately, we have been focusing on reducing the computational time needed to run such trip planners and managed to reach a reduction in the average execution time of about 91 percent (from about 80s to about 7s). However, when it comes to sharing data, other concerns must be taken into account, like privacy issues and the will to share such data among different stakeholders,” says Lorenzo Montalto.